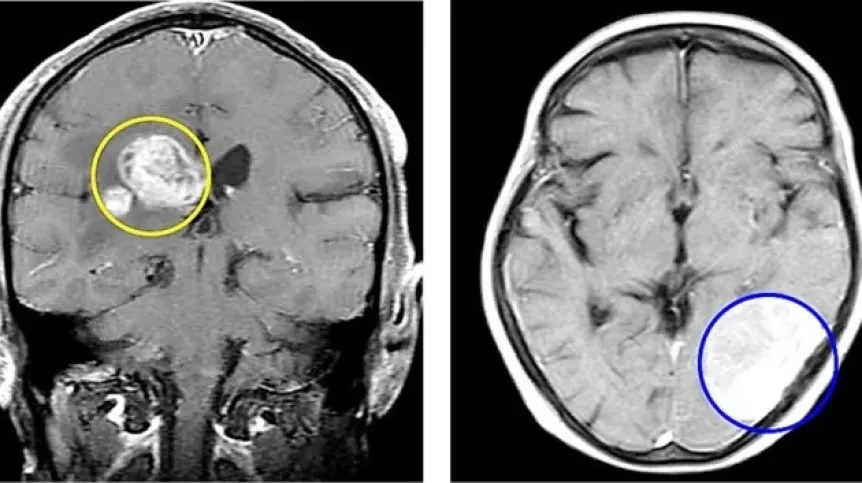
Scientists propose a new approach to data analysis that combines convolutional neural networks and extreme learning machines. This solution will enable early detection and precise classification of brain tumours. The new method uses magnetic resonance images.
The research on this topic was conducted by an international team with the participation of Marcin Kowalski, PhD, from the Institute of Optoelectronics of the Military University of Technology, the university reports.
The co-author of the new method explains that it can help medics and medical clinics to analyse patient tests faster. The doctor will be relieved of performing initial diagnostics and instead focus on developing the patient's treatment process.
DIAGNOSIS PROPOSAL PLUS JUSTIFICATION
According to the scientist from the Military University of Technology, the method has a chance of practical application, although it is computationally demanding (this reservation was also included in the text of the publication). The use of algorithms in diagnostics is one of the dominant topics of medical symposia.
'Brain tumours are a serious global health challenge, and their early detection and accurate classification are crucial for effective treatment strategies. If we hear that there are too few doctors in relation to the number of patients and the amount of work, why not use technology to streamline this process? Especially that the technology is present, quite well available and we can create it ourselves. In addition, medical centres have both the knowledge and data that we can use to create algorithms', Kowalski says.
Using the proposed method, the doctor would receive not only a diagnosis proposal, but also information on the basis on which the algorithm made the decision. This would include an image with marked areas and the result of the analysis. On this basis, the doctor would be able to make a decision in the context of further treatment.
'The basic objects that we wanted to distinguish in the images were glioma, meningioma, pituitary gland and of course the lack of tumour. The method showed incredible efficiency in image classification, reaching over 99 percent for the tested set', Kowalski summarises.
He adds that the sets the researchers used were limited in quantity and did not contain other patient metadata regarding, for example, genetic burden. Therefore, the study can be expanded by combining many pieces of information from different sources.
ALGORITHMS WILL 'EXTRACT' THE MOST IMPORTANT INFORMATION FROM THE IMAGE
Convolutional neural networks (CNNs) are a type of neural network, usually multi-layered, ideally suited for working with data in the form of images or other spatial data (e.g. 2D signals). Convolutional networks can be used for various purposes, from discriminant feature extractors to classifiers.
'Discriminant features are variables (attributes) that have the greatest power in differentiating between groups or classes. These data are crucial for the correctness of the classification model. By +extracting+ discriminant features from images, we can teach the classification algorithm to distinguish between an image showing a patient with and without a tumour', Kowalski explains.
On the other hand, extreme learning machines (ELMs) are algorithms developed for single-layer neural networks that focus on the speed of learning. 'In our work, we used convolutional networks to extract discriminant features (the most important information) from brain images, while an ELM, quite heavily modified, was used for classification, i.e. the operation of distinguishing between a healthy and diseased organ based on data extracted from images thanks to the use of CNN', he clarifies.
Convolutional networks and ELMs are used to extract discriminant features from images obtained in magnetic resonance imaging (MRI).
The results of the newly developed method will be interpreted by SHAP (SHapley Additive exPlanations). This algorithm allows scientists to understand on what basis the classification algorithm has made the decision on detecting a brain tumour.
'This is most often done by marking the appropriate areas on which the classification algorithm focused during the analysis of a given image. In other words, if SHAP (or another equivalent) were omitted, we would have the examined image and the analysis result without any additional information on how this result was achieved', Kowalski says.
A CHANCE TO REALLY IMPROVE HELP FOR THE PATIENTS
The researcher reminds that statistics from the World Health Organization (WHO) show that in 2018, cancer claimed 9.6 million lives worldwide. Brain tumours have become a significant factor contributing to this phenomenon.
Primary and secondary metastatic tumours pose distinct diagnostic and therapeutic challenges. Primary brain tumours arise within the brain, while secondary metastatic tumours stealthily infiltrate the brain via the bloodstream from other regions of the body.
The WHO divides brain tumors into four categories, which are classified according to their malignant or benign nature, spanning the spectrum from insidious growth to aggressive malignancies.
Marcin Kowalski's role in the project was to select and consult on the research methods, and analyse the results of the developed method. The key competences for the team's work included data preparation and pre-processing, method selection, programming, and data analysis. Hence the large group of authors of the paper published in the journal Scientific Reports.
PAP - Science in Poland, Karolina Duszczyk
kol/ zan/
Gallery (4 images)
-
1/4Brain MRI showing a glioma. Credit: Marcin Kowalski/Military University of Technology
-
2/4Brain MRI showing a meningioma. Credit: Marcin Kowalski/Military University of Technology
-
3/4Brain MRI showing the pituitary gland. Credit: Marcin Kowalski/Military University of Technology
-
4/4Brain MRI showing no tumors. Credit: Marcin Kowalski/Military University of Technology