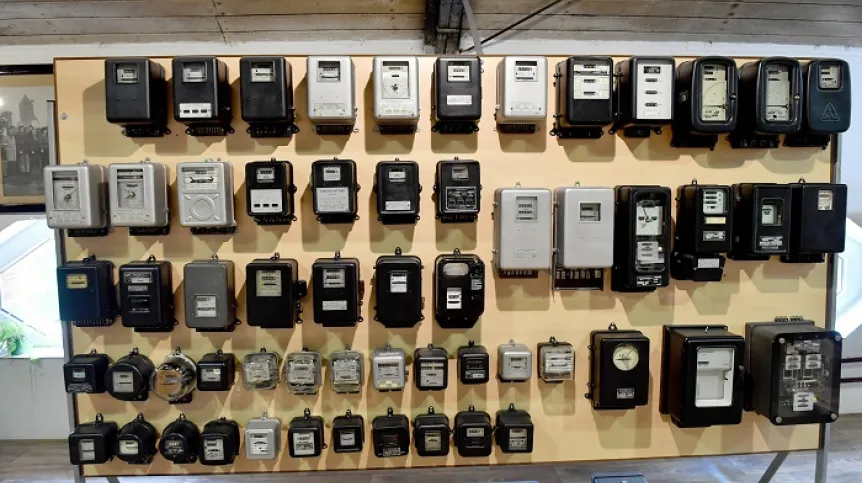
Scientists in Poland and the US have come up with a model for forecasting electricity prices to a high degree of accuracy.
Presented in the International Journal of Forecasting, the model named NBEATSx is more accurate than the best next day market price forecasting methods currently used by the electric power industry.
The authors of the paper are Professor Rafał Weron and his doctoral candidate Grzegorz Marcjasz from the Department of Operations Research and Business Intelligence of the Wrocław University of Science and Technology and scientists from the School of Computer Science at Carnegie Mellon University in the USA.
Professor Weron told PAP that scientific research in this area is of great practical importance. According to the estimates he cites, for a medium-sized power plant with an annual peak demand of 5 GW, improving next day price forecasts by 1 percent can lead to annual savings of $ 1.5 million.
He said: “In the past, energy companies used short-term demand forecasts to ensure the security of energy supply, and long-term forecasts to plan new investments.
“However, the financial costs of errors leading to later resale or purchase of energy in the balance market are so high that they can lead to bankruptcy.
“As a result, electricity forecasting prices and demand has become a central process in managing almost every major enterprise, not only an energy company.”
PRICES CAN GO CRAZY
He added: “While forecasting electricity demand is relatively simple, forecasting prices with the same accuracy is not. Especially for very short periods, not exceeding 48 hours, prices can go crazy. Very early in the morning, when the demand is low, they can be negative, only to jump sky-high in the afternoon.”
Because increasingly complex models are needed to forecast energy prices, to better represent the relationships between variables, they must take into account the growing amount of flowing data, including the data on expanding renewable energy sources (RES).
WHEN THE MODEL SURPASSES THE HUMAN MIND
According to Professor Weron, machine learning methods are now necessary to increase the amount of input data, which means scientists and practitioners are less and less involved not only in data processing, but even in the construction of the model itself.
He said: “This approach leads to more accurate forecasts, but it also has its disadvantages. We are no longer in control of the model, we cease to understand how it works. That is why in recent years scientists have started working on models that, despite their complexity, enable the interpretation of results.”
In 2019, Oreshkin, Carpov, Chapados and Bengio proposed a new deep neural network architecture. They named it the Neural Basis Expansion Analysis for Time Series (NBEATS). It is capable of time-series signal decomposition, and thus enables the interpretation of how individual fragments of the network work.
But despite the advantages of NBEATS and the great results that the method has achieved for data from the M4 forecasting competition, the NBEATS architecture is not suitable for forecasting electricity prices.
The idea behind Professor Weron’s team’s method consists of adding additional blocks to the NBEATS structure, allowing it to incorporate exogenous variables, for example forecasts for electricity demand or renewable energy generation. This makes it possible to model the effects caused by these two key factors. The result is the NBEATSx architecture, where "x" stands for exogenous variables.
The authors have shown that for electricity prices, their architecture is almost 20 percent more accurate than NBEATS and the ESRNN method, which won the M4 competition, and up to 5 percent more accurate than the best price forecasting methods.
PAP - Science in Poland, Karolina Duszczyk
kol/ zan/ kap/
tr. RL